Rahul Chowdhary, Kundan S Chufal, Irfan Ahmad, Akanksha Chhabra, M Jwala, Anjali Kakria, Maithili Sharma, Munish Gairola
Nov 1, 2020
This research paper aimed to develop prognostic models for patients treated for thymic epithelial tumors using Machine Learning (ML) and Artificial Neural Networks (ANN). The study included 60 patients with thymic epithelial tumors and collected demographic, clinical, and treatment-related variables. Variable selection for ANN modeling was performed using Two-Step Clustering (TSC) and Principal Component Analysis (PCA). The selected variables were used as input for ANN-based prognostic modeling, with overall survival as the outcome. The accuracy of the models was assessed using Area Under Curve (AUC) analysis.
TSC identified six variables (margin status, radiotherapy, stage, ECOG PS, histology, and volume) that significantly split the cohort into two groups with different median overall survival. MLP modeling with TSC-based feature selection achieved an overall accuracy of 92.9% in the training cohort and 87.5% in the validation cohort, with an AUC of 0.92.
PCA identified five variables (stage, surgery, margin status, sex, and duration of symptoms) that, when combined with histology and radiotherapy delivery, resulted in an MLP model with an overall accuracy of 84.2% in the training cohort and 87.0% in the validation cohort, with an AUC of 0.90.
The study concluded that prognostic modeling using ANN is feasible even with limited datasets and is well-suited for analyzing rare tumors. However, TSC-based ANN modeling led to overfitting, while PCA-based variable selection provided more accurate results.
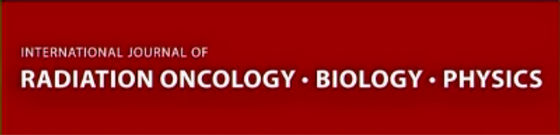